How Speech Translation works- Automatic Speech Recognition
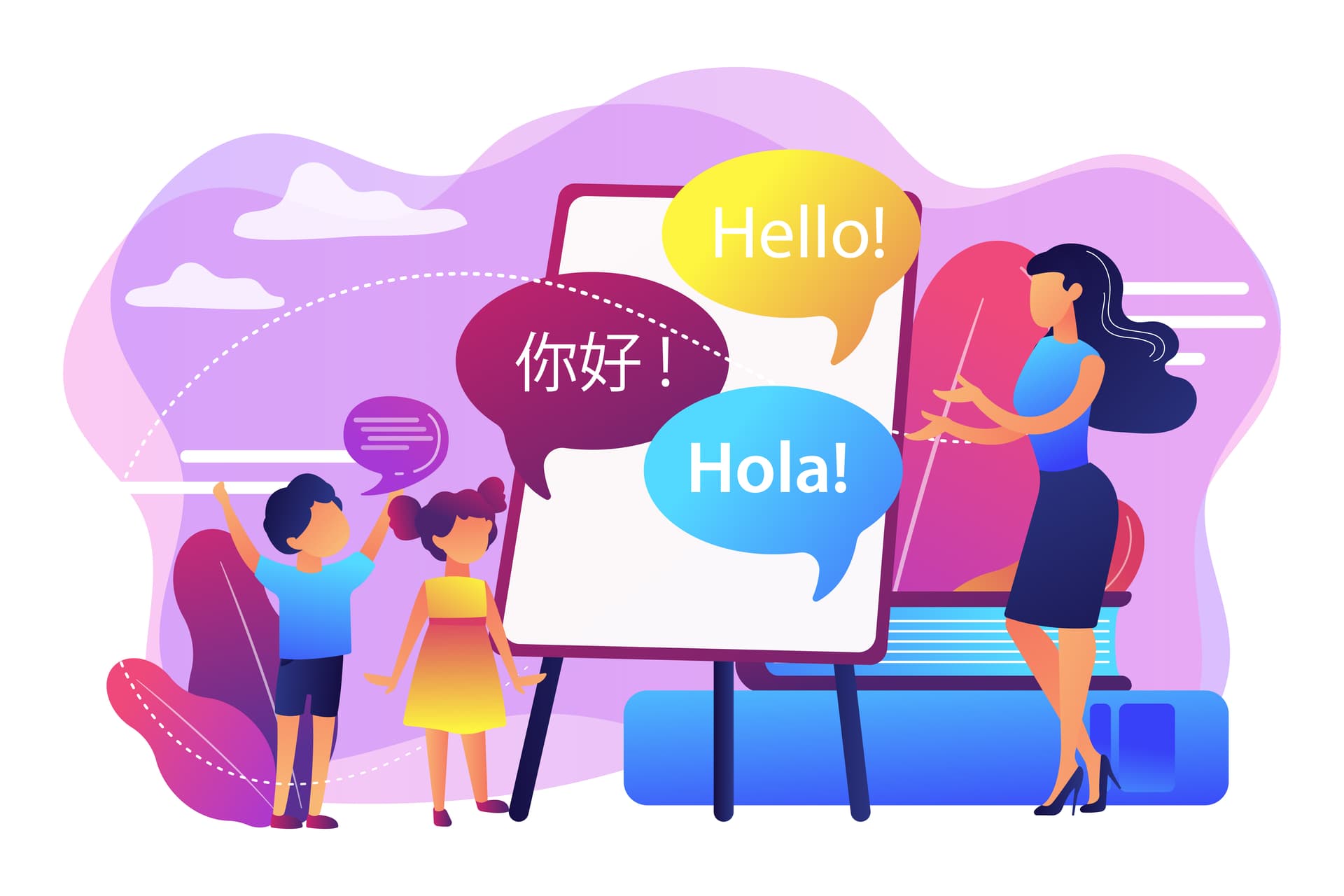
Speech translation converts spoken conversational words from one language to another, enabling seamless communication between people who speak different languages.
Process Flow
A simplified break-down of the process looks like below
- Automatic Speech Recognition: It will help in converting the spoken words & phrases into the text in the same language.
- Machine Translation: It will help in converting the text into a second language. It will replace each word in the text with the appropriate word in the second language.
- Speech Synthesis: It will estimate the pronunciation of the text generated by machine translation and generate the speech in the second and desired language.
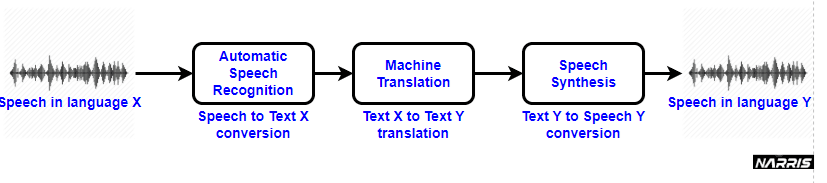
Image 1: High Level Process Flow of Speech Translation
Scope of this article is Automatic Speech Recognition.
What is Automatic Speech Recognition?
When we say speech recognition, we’re talking about ASR or automatic speech recognition, in which for any continuous speech audio input, the corresponding output text should be generated.
Why Signal Analysis is Required in Automated Speech Recognition
Signal analysis is essential in automated speech recognition (ASR) for several fundamental reasons:
- Speech-to-Data Conversion: Human speech exists as complex sound waves, but computers require numerical representations to process information. Signal analysis transforms these analog sound waves into digital representations that algorithms can understand.
- Feature Extraction: Raw audio waveforms contain excessive data, much of which is irrelevant for speech recognition. Signal processing extracts the most salient features that actually distinguish different speech sounds.
- Noise Reduction: Real-world audio contains background noise, reverberations, and distortions. Signal analysis helps separate speech from these unwanted elements.
- Pattern Recognition: Speech recognition requires identifying patterns across different speakers, accents, and acoustic environments. Signal analysis reveals consistent patterns in speech that remain stable across these variations.
- Efficiency: By transforming raw audio into optimized representations, signal analysis allows ASR systems to operate with manageable computational requirements.
Key Signal Analysis Techniques
1. Fourier Transform
The Fourier Transform is a mathematical technique that decomposes a time-domain signal (amplitude over time) into its constituent frequencies. It's based on the principle that any complex waveform can be represented as a sum of simple sine waves of different frequencies, amplitudes, and phases.
In ASR, Fourier Transform is crucial because:
- Human speech consists of different frequencies that correspond to distinct phonetic elements
- The frequency composition of speech sounds is more discriminative than time-domain representations
- It reveals the harmonic structure of speech, which helps identify vowels and voiced consonants
2. Fast Fourier Transform (FFT)
The Fast Fourier Transform is an efficient algorithm for computing the Discrete Fourier Transform (DFT), which is the practical implementation of Fourier analysis for digital signals.
Key aspects of FFT in ASR:
- Dramatically reduces computational complexity from O(n²) to O(n log n)
- Makes real-time speech processing feasible.
- Operates on short time windows (frames) of speech, typically 20-40ms.
- Forms the foundation for most speech feature extraction methods
The improvement in computational efficiency comes from exploiting symmetries and redundancies in the DFT calculation, particularly by recursively breaking down the calculation into smaller DFTs.
3. Spectrogram
A spectrogram is a visual representation of the spectrum of frequencies in a signal as they vary with time. It's essentially a sequence of FFTs computed over successive time windows of the speech signal.
In a spectrogram:
- The horizontal axis represents time
- The vertical axis represents frequency
- Color or brightness indicates amplitude/energy at each time-frequency point
Spectrograms are particularly valuable in ASR because:
- They visualize how speech frequencies change over time
- They reveal formants (resonant frequencies) that distinguish different vowels
- They show transitions between phonemes
- They help identify speech features even in noisy environments
- They form the basis for many advanced feature extraction techniques like MFCCs (Mel-Frequency Cepstral Coefficients)
Modern ASR systems often use spectral representations as inputs to neural networks, which learn to recognize patterns in these time-frequency representations that correspond to words and phrases.
Architecture
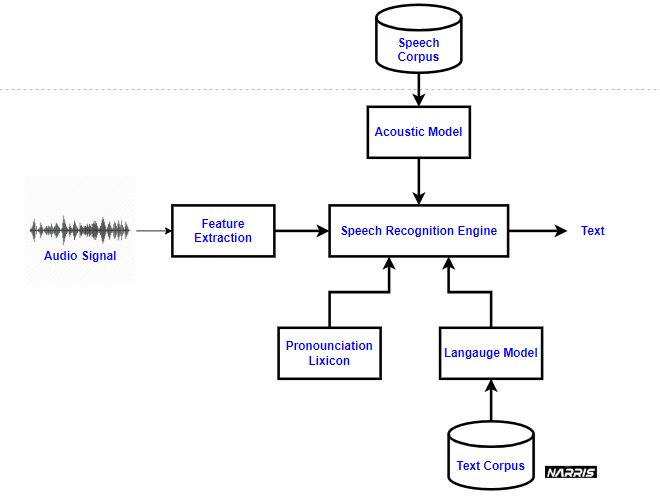
Image 2: Technical Architecture of ASR
- Speech Signal: The process begins with a speech signal, which is the audio input of someone speaking.
- Feature Extraction: The speech signal is analyzed, and relevant features are extracted. This step transforms the audio signal into a set of features that represent the essential characteristics of the speech for further processing.
- Speech Recognition Engine: The extracted features are fed into the speech recognition engine. This is the core component that processes the features to identify the words spoken.
- Acoustic Model: The speech recognition engine utilizes an acoustic model, which is trained on a speech corpus. This model helps understand how different phonetic sounds correspond to specific textual representations.
- Pronunciation Lexicon: The pronunciation lexicon provides information on how words are pronounced. It assists the acoustic model by mapping the features to the correct phonetic sounds.
- Language Model: This model helps in predicting the likelihood of a sequence of words. It uses a text corpus to understand context and improve the accuracy of the transcription.
- Output Text: After processing through the various models (acoustic model, pronunciation lexicon, and language model), the speech recognition engine generates the final output in text format.
Key Algorithms & Techniques in Speech Recognition
Speech recognition relies on various algorithms and computational techniques to convert speech into text with high accuracy. Below are some of the most commonly used methods:
1️. Natural Language Processing (NLP)
- Focuses on human-machine interaction through speech and text.
- Enhances applications like voice search (e.g., Siri) and text accessibility features.
2. Hidden Markov Models (HMMs)
- Based on Markov chains, where the probability of a state depends only on the current state.
- Used in speech recognition to map audio sequences to text, assigning probabilistic labels to words, syllables, or sentences.
3️. N-Grams (Language Models)
- Assign probabilities to word sequences to enhance recognition accuracy.
- Example: "order the pizza" (trigram), "please order the pizza" (4-gram).
4️. Neural Networks (Deep Learning)
- Mimic the human brain using layers of interconnected nodes.
- Improve accuracy through supervised learning and gradient descent but require high computational resources.
5️. Speaker Diarization (SD)
- Identifies and segments speakers in a conversation.
- Used in call centers to distinguish between customers and agents.
Technical Flow
- Speech Input & Feature Extraction: Speech signal is captured and converted into feature vectors using acoustic-prosodic features such as pitch, energy, and spectral content. Following techniques are used:
- Mel-Frequency Cepstral Coefficients (MFCCs)
- Spectrogram Analysis
- Phoneme Segmentation: The speech waveform is segmented into phonemes, which are the smallest units of speech. Example:The word "world" is broken down into phonemes /w ɜːr l d/.
- Hidden Markov Model (HMM) Training: Each word is modeled as an HMM, where:
- States represent phonemes.
- Observations correspond to feature vectors extracted from speech.
- Emission Probabilities → Probability of observing a feature given a hidden phoneme state.
- Transition Probabilities → Probability of moving from one phoneme state to another.
Example: HMM for the word "world" has 5 states, each representing a phoneme, with probabilities estimated from training data. - Speech Recognition using HMMs: Given a new speech input, the system calculates the likelihood of the input belonging to each HMM and selects the word with the highest probability.
- Extract feature vectors from speech.
- Compare with stored HMM probabilities.
- Select the word with the highest likelihood.
- Decoding with the Viterbi Algorithm: The Viterbi Algorithm is used to determine the most probable sequence of phonemes or words.
- Initialize the Viterbi matrix to store max likelihood of each state at each time step.
- Compute likelihood scores for each phoneme transition.
- Track the most probable path using a backpointer matrix.
- The final state with the highest probability is selected as the recognized word.
- Language Model Integration (N-Gram Models): The N-Gram Language Model improves accuracy by predicting word sequences based on previous words.
Examples:- Unigram: "world"
- Bigram: "world is"
- Trigram: "world is one"
Challenges of N-Grams:- Data sparsity → Rare word combinations may have zero probability.
- Computational cost → Higher N values require more memory and processing power.
- Lack of context → N-grams only consider limited word history.
- Speaker Diarization (Who Spoke When?): Speaker diarization separates multiple speakers in an audio recording.
Techniques Used:- Clustering → Groups speech segments with similar acoustic features.
- Classification → Machine learning models assign speech segments to specific speakers.
- Decoding → Uses HMMs or Deep Neural Networks (DNNs) to infer speaker identity..
Challenges in Speech Recognition
There are a few challenges involved in developing speech recognition models
Narris provides secure, scalable pipelines designed to support high-performance, robust, and custom deep learning models for addressing the complexities of Automatic Speech Recognition (ASR). Our infrastructure ensures efficiency, accuracy, and resilience, enabling seamless processing of speech data at scale. With advanced AI-driven optimizations, Narris delivers state-of-the-art solutions to tackle ASR challenges with precision and reliability.